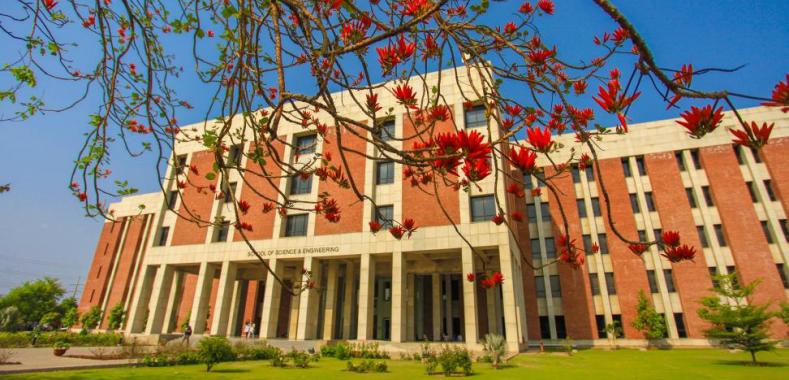
On October 25, 2019, the Department of Electrical Engineering at the Syed Babar Ali School of Science and Engineering held a talk on ‘On-Chip Chronic Disease Monitoring: Where Machine Learning Meets Patient-Specific Wearable Healthcare Applications’. The session was led by Dr. Muhammad Awais Bin Altaf, Assistant Professor LUMS.
Dr. Altaf received his Masters and PhD degrees in microsystems engineering and interdisciplinary engineering from the Masdar Institute of Science and Technology (MIST), UAE. During his time at MIST, he developed an energy-efficient machine-learning based feature extraction and classification processor as well as a System-on-Chip (SoC) for epileptic seizure detection. He is the recipient of the IEEE Solid-State Circuits Society Predoctoral award for his work on efficient machine learning hardware implementation for wearable healthcare in 2016. His current research interests include physiological signal monitoring, breast cancer detection, and the development of low-power mixed-signal circuits for portable medical applications.
The topic was especially relevant given that early detection of chronic diseases can help in reducing mortality, slowing disease progression, and mitigating complications to optimise the quality of life and decrease the demand on the healthcare system. Detecting chronic diseases from physiological signals such as electroencephalogram (EEG) or electrocardiogram (ECG) is challenging due to each patient's abnormal pattern being very different from each other. To detect such abnormal patterns in real time, the system must learn each patient's ictal pattern from the physiological signal traces through machine learning approaches, hence achieving a patient-specific disease detection. Since this new class of biomedical systems becomes more ubiquitous, the design and development of energy-efficient techniques gain more significance to allow a sustainable realisation of the entire monitoring system.
The talk provided an overview of the design strategies of energy-efficient patient-specific SoC biomedical devices. It also explored the challenges, limitations and potential pitfalls in wearable interface circuit design, and the strategies to overcome such issues. Dr. Altaf described on-chip energy-efficient digital processing techniques for the implementation of machine learning algorithms for disease detection focusing on two examples: reduced feature set early detection of ventricular arrhythmia processor, and a portable breast-cancer early screening device.
The talk concluded with a discussion around the interesting aspects of the field and the opportunities that lie ahead.