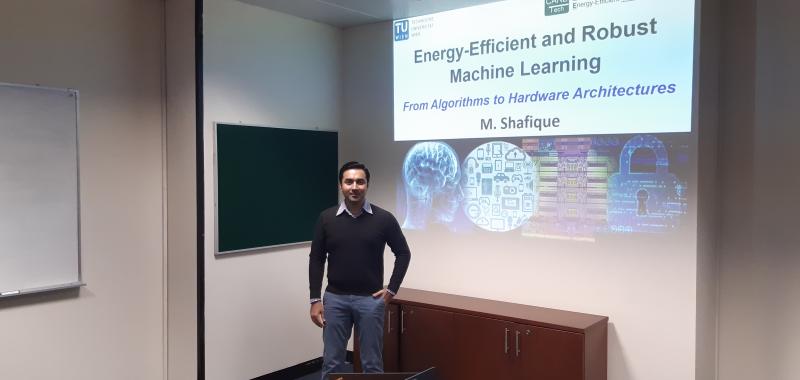
The Department of Electrical Engineering at the Syed Babar Ali School of Science and Engineering (SBASSE) held a talk on 'Energy-Efficient and Robust Machine Learning: From Algorithms to Hardware' on December 24, 2019. Dr. Muhammad Shafique, Professor at the Institute of Computer Engineering, Faculty of Informatics, Vienna University of Technology, Austria presented the talk.
The talk discussed the gigantic rates of data production in the era of Big Data, Internet of Thing (IoT), and Smart Cyber Physical Systems (CPS) pose incessantly escalating demands for massive data processing, storage, and transmission while continuously interacting with the physical world under unpredictable, harsh, and energy-constrained scenarios. Therefore, such systems need to support not only the high-performance capabilities at tight power/energy envelop, but also need to be intelligent/cognitive and robust. This has given rise to a new age of Machine Learning (and, in general Artificial Intelligence) at different levels of the computing stack, ranging from Edge and Fog to the Cloud. In particular, Deep Neural Networks (DNNs) have shown a tremendous improvement over the past 5-7 years to achieve a significantly high accuracy for a certain set of tasks, like image classification, object detection, and natural language processing. However, these DNN require highly complex computations, costing a huge amount of processing, memory, and energy budgets. To some extent, Moore’s Law help by packing more transistors in the chip. However, at the same time, every new generation of device technology faces new issues and challenges in terms of energy efficiency, power density, and diverse reliability threats. These technological issues and the escalating challenges posed by the new generation of IoT and CPS systems force to rethink the computing foundations, architectures and the system software. Moreover, in the era of growing cyber-security threats, the intelligent features of a smart CPS and IoT system face new type of attacks, requiring new design principles for enabling Robust Machine Learning.
“At the Caretech Group in the Vienna University of Technology (TU Wien) Austria, we are investigating the foundations for the next-generation energy-efficient and robust computing systems while addressing the above-mentioned challenges across hardware and software stacks. I have presented different design challenges for building highly energy-efficient and robust machine learning systems, covering both the efficient software and hardware designs,” shared Dr. Shafique.
After presenting a quick overview of state-of-the-art design trends, Dr. Shafique presented the research roadmap and early results from his Brain-Inspired Computing project, ranging from approximate computing to neural processing with specialised machine learning hardware, covering both fundamental and technological challenges, which enable new opportunities for improving the area, power/energy, and performance efficiency of systems by orders of magnitude. He also shared a quick overview of different reliability and security aspects of the machine learning systems deployed in Smart CPS and IoT.
The talk presented a cross-layer design flow for machine learning (and in particular for DNNs), that jointly leverages efficient optimizations at different software and hardware layers, is a crucial step towards enabling the wide-scale deployment of DNNs in resource-constrained embedded AI systems like UAVs, autonomous vehicles, Robotics, IoT-Healthcare / Wearables, Industrial-IoT, etc.