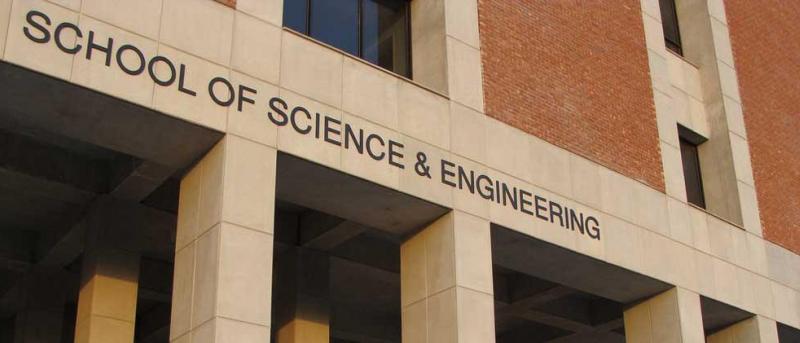
PhD Dissertation Defense of Computer Science student, Mubasher Baig
Title: Boosting Based Multiclass Ensembles and Their Application in Machine Learning
Date: Wednesday, December 28, 2016
Time: 11:00 am
Venue: CS Smart Lab, Ground floor, SBASSE
Evaluation Committee
Mian M Awais (Advisor, CS Department, LUMS)
Asim Karim (Member, CS Department, LUMS)
Shafay Shamail (Member, CS Department, LUMS)
Ahmad Kamal Nasir (Member, EE Department, LUMS)
Kashif Javed (External Examiner, EE Department, UET, Lahore)
The abstract of the thesis and list of publications is given below
Abstract
Boosting is a generic statistical process for generating accurate classifier ensembles from only a moderately accurate learning algorithm. AdaBoost (Adaptive Boosting) is a machine learning algorithm that iteratively fits a number of classifiers on the training data and forms a linear combination of these classifiers to form a final ensemble. This dissertation presents our three major contributions to boosting based ensemble learning literature which includes two multi-class ensemble learning algorithms, a novel way to incorporate domain knowledge into a variety of boosting algorithms and an application of boosting in a connectionist framework to learn a feed-forward artificial neural network. To learn a multi-class classifier a new multi-class boosting algorithm, called M-Boost, has been proposed that introduces novel classifier selection and classifier combining rules. M-Boost uses a simple partitioning algorithm (i.e., decision stumps) as base classifier to handle a multi-class problem without breaking it into multiple binary problems. It uses a global optimality measures for selecting a weak classifier as compared to standard AdaBoost variants that use a localized greedy approach. It also uses a confidence based reweighing strategy for training examples as opposed to standard exponential multiplicative factor. Finally, M-Boost outputs a probability distribution over classes rather than a binary classification decision. The algorithm has been tested for eleven datasets from UCI repository and has consistently performed much better for 9 out of 11 datasets in terms of classification accuracy. Another multi-class ensemble learning algorithm, CBC: Cascaded Boosted Classifiers, is also presented that creates a multiclass ensemble by learning a cascade of boosted classifiers. It does not require explicit encoding of the given multiclass problem, rather it learns a multi-split decision tree and implicitly learns the encoding as well. In our recursive approach, an optimal partition of all classes is selected from the set of all possible partitions and training examples are relabeled. The reduced multiclass learning problem is then learned by using a multiclass learner. This procedure is recursively applied for each partition in order to learn a complete cascade. For experiments we have chosen M-Boost as the multi-class ensemble learning algorithm. The proposed algorithm was tested for network intrusion detection dataset (NIDD) adopted from the KDD Cup 99 prepared and managed by MIT Lincoln Labs as part of the 1998 DARPA Intrusion Detection Evaluation Programme.
To incorporate domain knowledge into boosting an entirely new strategy for incorporating prior into any boosting algorithm has also been devised. The idea behind incorporating prior into boosting in our approach is to modify the weight distribution over training examples using the prior during each iteration. This modification affects the selection of base classifier included in the ensemble and hence incorporate prior in boosting. Experimental results show that the proposed method improves the convergence rate, improves accuracy and compensates for lack of training data.
A novel weight adaptation method in a connectionist framework that uses AdaBoost to minimize an exponential cost function instead of the mean square error minimization is also presented in this dissertation. This change was introduced to achieve better classification accuracy as the exponential loss function minimized by AdaBoost is more suitable for learning a classifier. Our main contribution in this regard is the introduction of a new representation of decision stumps that when used as base learner in AdaBoost becomes equivalent to a perceptron. This boosting based method for learning a perceptron is called BOOSTRON. The BOOSTRON algorithm has also been extended and generalized to learn a multi-layered perceptron. This generalization uses an iterative strategy along with the BOOSTRON algorithm to learn weights of hidden layer neurons and output neurons by reducing these problems into problems of learning a single layer perceptron.
Publications
Conference Papers
1) Mirza Mubasher Baig, El-Sayed M. El-Alfy, Mian M. Awais:Learning Rule for Linear Multilayer Feedforward ANN by Boosted Decision Stumps. ICONIP 2015: 345-353. Core Rating A.
2) Mirza Mubasher Baig, Mian M. Awais, El-Sayed M. El-Alfy: BOOSTRON: Boosting Based Perceptron Learning. ICONIP 2014:199-206. Core Rating A.
3) Mirza Mubasher Baig, El-Sayed M. El-Alfy, Mian M. Awais: Intrusion detection using a cascade of boosted classifiers (CBC). IJCNN 2014: 1386-1392. Core Rating A.
4) Mirza Mubasher Baig, Mian Muhammad Awais:Global Reweighting and Weight Vector Based Strategy for Multiclass Boosting. ICONIP 2012: 452-459. Core Rating A.
Journal paper
1) Mirza Baig, Mian Muhammad Awais and El-Sayed M El-Alfy: A Multiclass Cascade of Artificial Neural Network for Network Intrusion Detection. Accepted for publication in Journal of Intelligent and Fuzzy Systems, IOS Press, Impact factor 1.004
Journal Papers under review
1) AdaBoost Based Artificial Neural Network Learning with manuscript number: Under review with Neurocomputing. NEUCOM-D-16-02115
2) Combining Boosting and Prior for Accurate Classification: Under review with International Journal of Pattern Recognition and AI.